Generative AI for Data Modeling: Simplified Insights
- Staff Desk
- Sep 11, 2024
- 5 min read
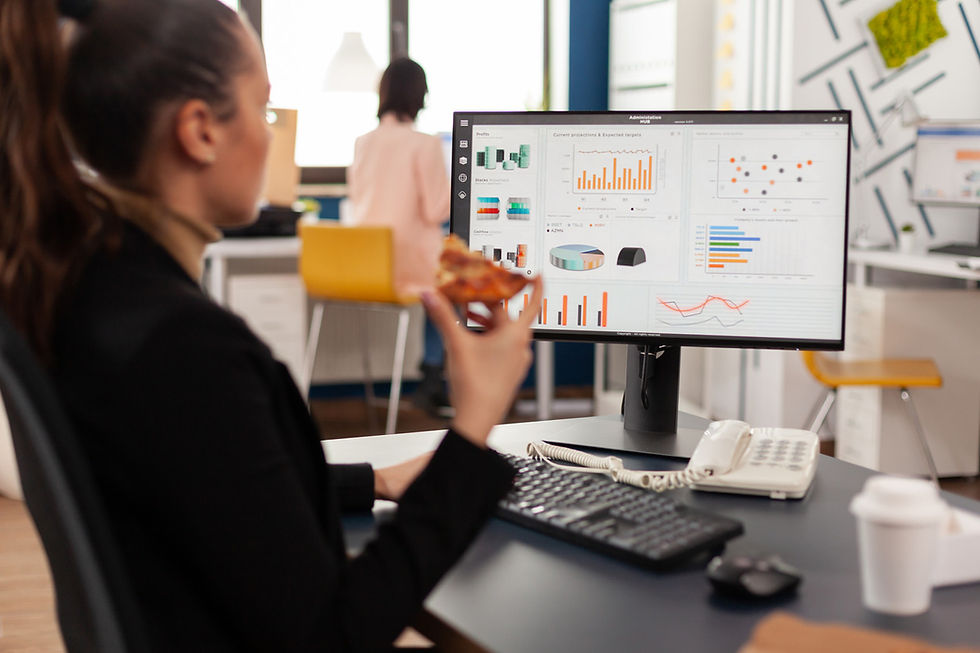
Generative AI improves predictive analytics by building models that can forecast future trends based on historical data. For instance, AI used in data analysis can create models to predict future outcomes. Generative AI, or foundation models, are built to produce various kinds of content, including text, chat, images, code, video, and embeddings. These models utilize neural networks to recognize patterns and structures in existing data, enabling them to generate new and original content.
Understand Generative AI?
Generative AI is a type of artificial intelligence that can create new data based on patterns it has learned from existing data. Think of it like a skilled artist who, after studying various art styles, can generate new paintings that resemble the ones they’ve studied but are original creations. In data modeling, Generative AI uses learned patterns to produce synthetic data that mirrors real-world data but isn't directly copied from it.
How Does Generative AI Work in Data Modeling?
Learning from Data: Generative AI starts by analyzing a large dataset to understand its underlying patterns, structures, and relationships. For instance, if you provide it with customer purchase data, the AI will learn about buying behaviors, seasonal trends, and product preferences.
Generating New Data: Once it has learned from the data, the AI can generate new, synthetic data that maintains the same characteristics as the original. This could be new customer profiles, simulated transaction logs, or hypothetical market conditions.
Refining Models: This generated data is then used to test and refine data models. For example, businesses can use synthetic customer data to simulate how changes in their strategies might impact sales, without needing access to actual customer data.
Why Use Generative AI for Data Modeling?
Enhanced Privacy: Since the generated data isn’t real, it can help protect sensitive information. For instance, if you’re working with personal data, you can use synthetic data to test systems and models without exposing real individuals’ details.
Increased Flexibility: Generative AI can produce vast amounts of synthetic data quickly. This is useful when real data is scarce or when you need diverse datasets to train your models effectively.
Improved Testing: By generating data that mimics various scenarios, businesses can better test their models under different conditions. This helps in understanding potential outcomes and making more informed decisions.
Cost Efficiency: Creating and maintaining large datasets can be expensive. Generative AI reduces the need for extensive data collection and management by creating synthetic datasets that are both cost-effective and versatile.
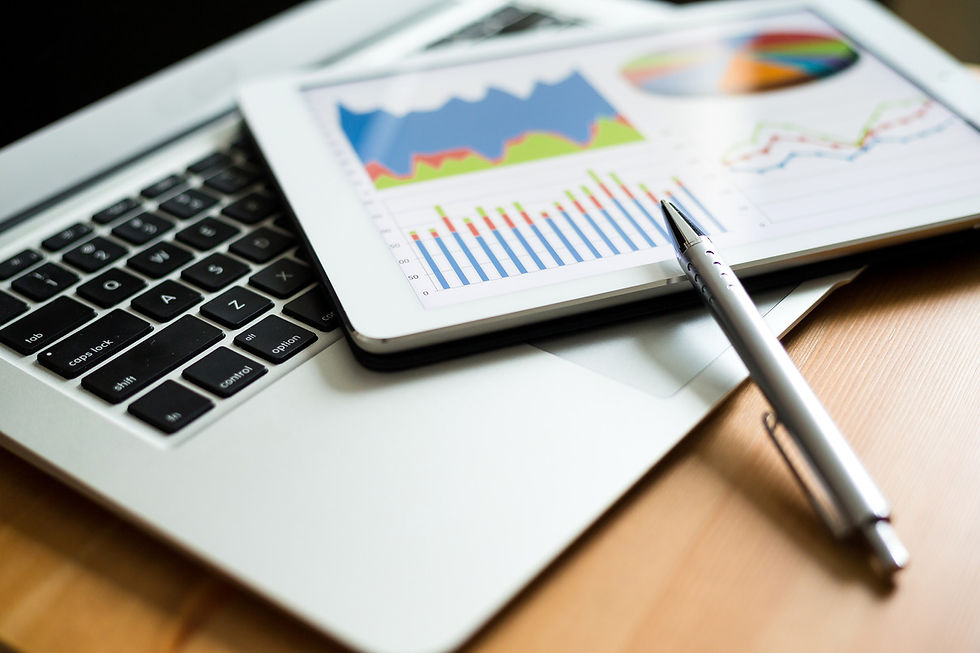
Practical Applications of Generative AI in Data Modeling
Healthcare: Generative AI can simulate patient data to predict disease outcomes and test new treatments without compromising patient privacy.
Finance: It can generate financial transaction data to test fraud detection systems or evaluate the impact of different financial strategies.
Retail: Retailers can use it to create synthetic customer data to optimize inventory, test marketing strategies, or enhance recommendation systems.
Automotive: In the automotive industry, generative AI can help simulate driving conditions to improve safety features and autonomous driving systems.
Specialties of Generative AI for Data Modeling
Advanced Data Simulation: Generative AI excels at creating detailed and realistic synthetic data, which can simulate a wide range of scenarios. This capability allows businesses to model complex situations and test various outcomes without relying solely on real-world data. For example, in healthcare, AI can simulate patient responses to different treatments, providing valuable insights for research and development.
Enhanced Privacy and Compliance: By generating synthetic data that mimics real-world data without containing actual personal information, Generative AI helps organizations adhere to privacy regulations and data protection laws. This is particularly crucial in industries such as finance and healthcare, where data sensitivity is paramount.
Rapid Data Generation and Scalability: Generative AI can quickly produce large volumes of synthetic data, which is beneficial when working with limited real data or when extensive datasets are required for training models. This scalability allows for more comprehensive model training and better performance in predictive analytics.
Cost-Effective Data Management: Generating synthetic data can be more cost-effective than collecting and maintaining large datasets. By reducing the need for extensive data collection and storage, organizations can save on expenses while still obtaining high-quality data for analysis and model development.
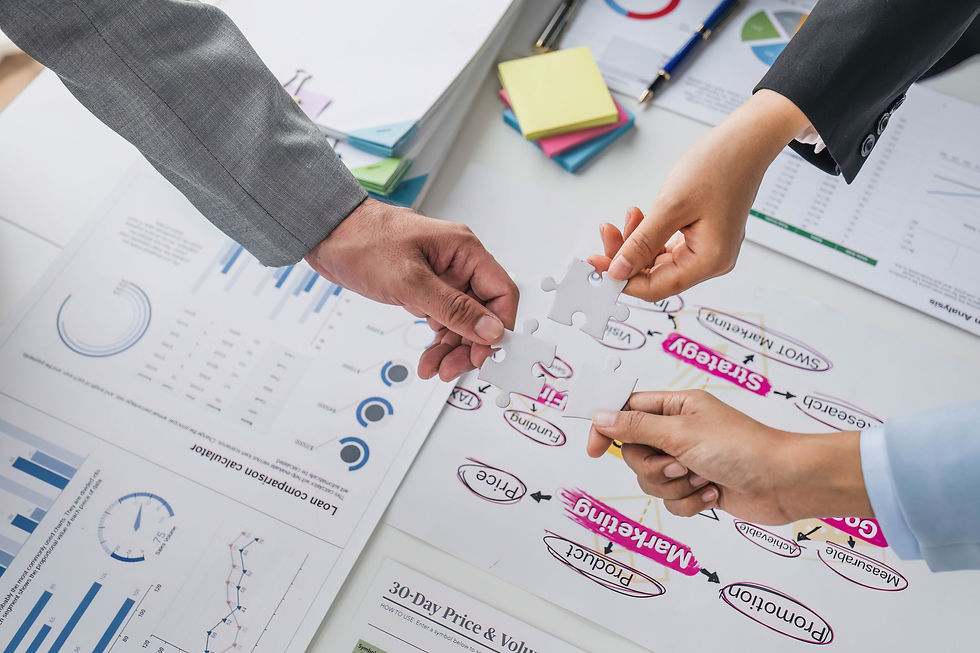
How Synlabs Help You
Innovative Technology Solutions: Partners are attracted to our advanced Generative AI capabilities that offer cutting-edge solutions for data modeling. Our technology enables businesses to leverage synthetic data for improved predictive analytics, leading to more accurate forecasting and strategic decision-making.
Commitment to Data Privacy: Our emphasis on privacy and data protection aligns with industry standards and regulations, making us a trusted partner for organizations concerned about data security. Our synthetic data solutions help partners maintain compliance with data privacy laws while still gaining valuable insights.
Scalable and Flexible Data Solutions: We provide scalable solutions that can quickly adapt to the evolving needs of our partners. Whether they require large volumes of synthetic data for training complex models or need specific data scenarios for testing, our flexible approach ensures that we meet their diverse requirements.
Cost Efficiency and Value: By offering cost-effective data generation and management solutions, we help our partners reduce expenses associated with data collection and storage. Our approach delivers high-value outcomes without the financial burden of traditional data management practices.
Footnote: Key Statistics
Data Privacy: A study by Gartner found that 72% of organizations are prioritizing data privacy and compliance as a major part of their data strategy in 2024.
Cost Savings: According to a report by McKinsey, companies using synthetic data can save up to 40% in data management costs compared to traditional data collection methods.
Data Generation: Research by MIT Technology Review indicates that Generative AI can produce synthetic data 10 times faster than manual data collection processes.
Predictive Accuracy: A survey by IBM found that businesses using AI for predictive analytics see a 20-30% improvement in forecast accuracy compared to those using traditional methods.
These statistics underscore the transformative impact of Generative AI on data modeling and highlight its potential benefits for organizations across various industries.
Conclusion
Generative AI represents a powerful advancement in data modeling, offering a host of benefits that transform how businesses approach predictive analytics. By learning from existing data and creating realistic synthetic data, Generative AI enhances predictive accuracy, improves data privacy, and provides a cost-effective solution for managing large datasets.
Through its ability to simulate complex scenarios and generate vast amounts of data quickly, Generative AI enables businesses to test models under varied conditions and gain insights that were previously challenging to obtain. This technology not only helps in refining data models but also ensures compliance with privacy regulations, making it invaluable in sensitive industries such as healthcare and finance.
Moreover, the cost efficiencies and scalability offered by Generative AI reduce the financial burden associated with data collection and management, delivering high-value outcomes without traditional constraints.
FAQs About Generative AI in Data Modeling
Is Generative AI only useful for large datasets?
Not necessarily. While it excels with large datasets, it can also be beneficial for smaller datasets by creating additional synthetic data to improve model performance and robustness.
How does Generative AI ensure the synthetic data is realistic?
Generative AI models are trained on real data, learning its patterns and structures. When generating synthetic data, these models replicate these patterns to ensure the data remains realistic and useful.
Can Generative AI replace real data?
No, Generative AI cannot replace real data entirely. However, it can supplement real data, especially when real data is limited, sensitive, or costly to obtain.
How do businesses ensure the quality of the synthetic data?
Quality is ensured by validating the synthetic data against real data and evaluating its performance in real-world scenarios. This involves comparing how well models trained on synthetic data perform compared to those trained on real data.
Are there any limitations to using Generative AI for data modeling?
Yes, limitations include the potential for generating data that may not perfectly capture rare or complex scenarios present in real data. Additionally, the effectiveness of generative models depends on the quality and representativeness of the data they are trained on.
Comments