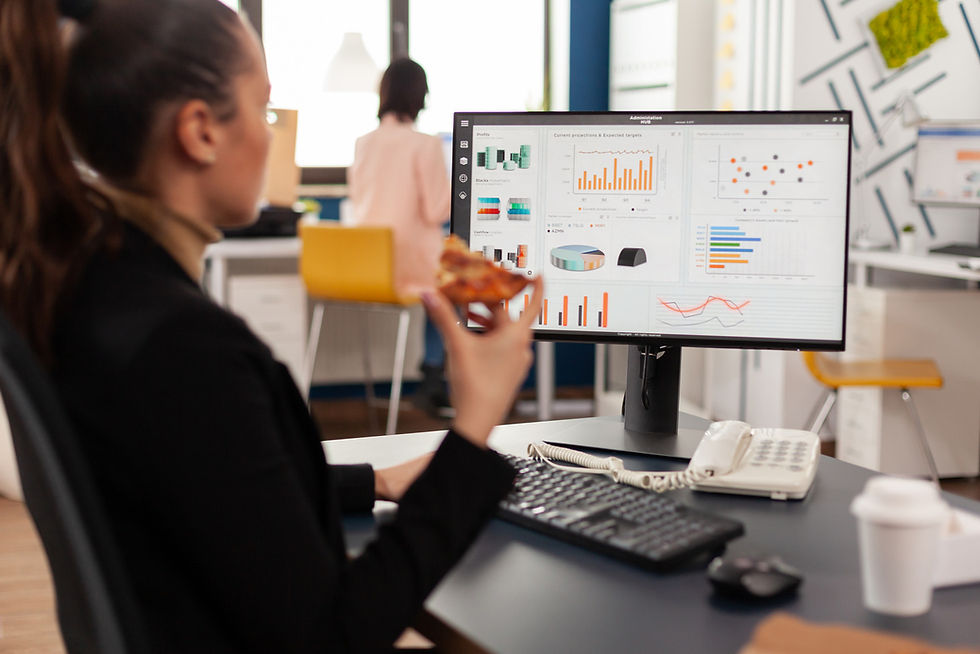
Leveraging generative AI with tabular data offers significant benefits for developers. It can transform how you make decisions, improve data privacy, enhance data quality, and support scalability. In practical terms, this means you can generate realistic data for model training, while also protecting sensitive information and ensuring your data practices are robust and scalable.
What is Generative AI for Tabular Data?
Generative AI refers to AI models designed to create new data based on existing patterns and structures. When applied to tabular data—data organized in rows and columns, such as spreadsheets or database tables—Generative AI can generate synthetic data, improve data quality, and provide advanced analytical capabilities. Unlike traditional methods, which focus on analyzing existing data, Generative AI can create new, realistic data points and scenarios that help businesses gain deeper insights and make more accurate predictions.
How Generative AI is Transforming Tabular Data
1. Synthetic Data Generation
Enhancing Data Privacy: One of the key applications of Generative AI in tabular data is the creation of synthetic data. This artificial data mimics real-world data but does not contain any personal or sensitive information. For industries dealing with privacy concerns—such as healthcare or finance—synthetic data allows for safe data sharing and collaboration while protecting individual privacy. According to a 2024 report by Deloitte, the use of synthetic data can reduce privacy risks by up to 75%.
Improving Data Availability: Generative AI can create additional data to fill gaps in existing datasets. This is particularly useful when dealing with rare events or underrepresented populations. For example, if a dataset lacks sufficient examples of a specific anomaly, Generative AI can simulate these anomalies, providing a more comprehensive dataset for training models and making predictions.
2. Data Augmentation and Enhancement
Augmenting Training Datasets: For machine learning models, having diverse and comprehensive training data is crucial. Generative AI can augment existing datasets by generating new samples that add variety and complexity. This augmentation helps in building more robust models that can generalize better to unseen data. A 2023 study published in Journal of Machine Learning Research found that data augmentation through Generative AI improved model accuracy by up to 30%.
Error Correction and Data Cleaning: Generative AI can assist in cleaning and correcting errors in tabular datasets. By learning the underlying patterns in the data, AI models can identify and rectify anomalies or inconsistencies, ensuring that the data used for analysis is accurate and reliable. This capability helps in maintaining the integrity of data-driven insights.
3. Advanced Data Analysis and Insights
Scenario Simulation: Generative AI can simulate various scenarios by generating new data based on different assumptions or conditions. This allows businesses to explore potential outcomes and make data-driven decisions. For instance, a retail company can use Generative AI to simulate different sales scenarios and predict how changes in pricing or inventory might impact revenue.
Predictive Modeling: Generative AI enhances predictive modeling by generating data that reflects future trends or potential changes. This helps businesses anticipate market shifts or operational challenges. A 2024 report by IBM highlighted that AI-driven predictive models could improve forecasting accuracy by up to 25%.
4. Personalization and Targeting
Customized Recommendations: Generative AI can analyze tabular data to create personalized recommendations for customers. By understanding individual preferences and behaviors, AI can generate tailored suggestions that enhance user experience and engagement. For example, e-commerce platforms use Generative AI to recommend products based on a user’s past purchases and browsing history.
Targeted Marketing: Businesses can use Generative AI to segment their customer base more effectively and design targeted marketing campaigns. By generating detailed customer profiles and predicting future behaviors, companies can create more precise and impactful marketing strategies.
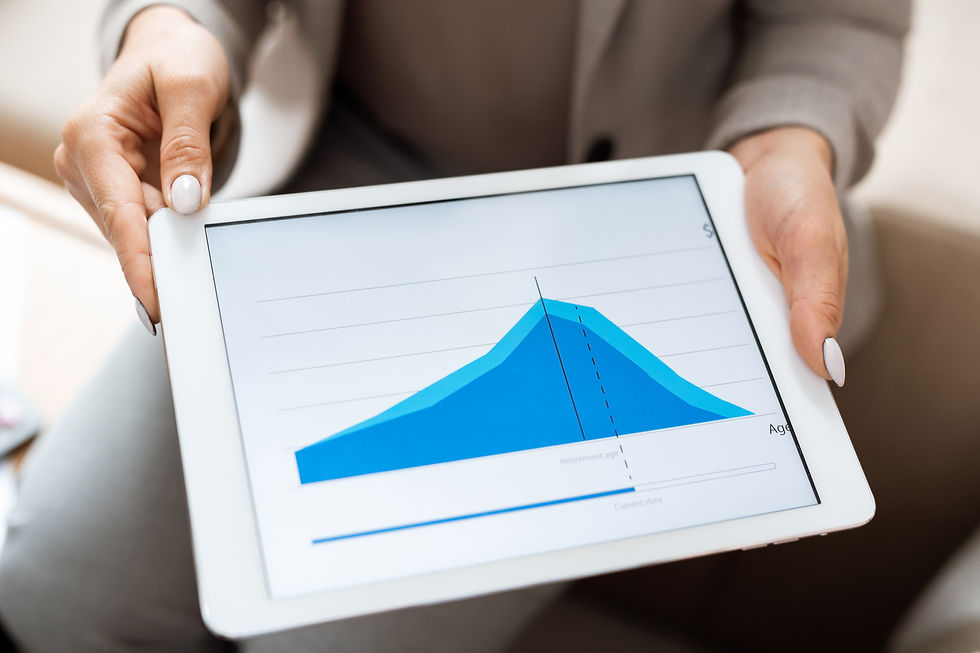
Frequently Asked Questions (FAQs)
1. What are the primary benefits of using Generative AI for tabular data?
Generative AI offers several benefits, including enhanced data privacy through synthetic data generation, improved data availability by filling gaps, better model accuracy through data augmentation, error correction, advanced scenario simulation, and more personalized recommendations. These advantages lead to more accurate insights, efficient data management, and improved decision-making.
2. How does Generative AI enhance data privacy?
Generative AI enhances data privacy by creating synthetic data that replicates the statistical properties of real data without including any personal or sensitive information. This synthetic data can be used for analysis and model training without compromising privacy, making it ideal for industries with stringent data protection requirements.
3. What is the role of Generative AI in data augmentation?
Generative AI plays a crucial role in data augmentation by generating additional data samples to enrich existing datasets. This process introduces new variations and complexities, which helps improve the performance and generalization of machine learning models, leading to more accurate predictions.
4. How does Generative AI assist in data cleaning and error correction?
Generative AI assists in data cleaning and error correction by learning the underlying patterns in the data and identifying anomalies or inconsistencies. By correcting these errors and inconsistencies, AI ensures that the data used for analysis is accurate and reliable, which enhances the quality of data-driven insights.
5. Can Generative AI help in predicting future trends?
Yes, Generative AI can help in predicting future trends by generating data that reflects potential future scenarios or changes. This capability allows businesses to explore different outcomes and make informed decisions based on simulated future trends, improving forecasting accuracy and strategic planning.
6. What are the challenges associated with implementing Generative AI for tabular data?
Challenges associated with implementing Generative AI for tabular data include the need for substantial computational resources, the risk of generating biased or unrealistic data if not properly managed, and the requirement for ongoing model training and validation. Addressing these challenges requires careful planning, investment in technology, and expertise.
7. How can businesses start integrating Generative AI into their data management processes?
Businesses can start integrating Generative AI by identifying specific use cases that align with their data needs, such as data augmentation or predictive modeling. They should invest in the necessary technology and expertise, pilot AI solutions, and scale up based on performance and results.
Conclusion
Generative AI is poised to revolutionize the management and analysis of tabular data. By generating synthetic data, enhancing data quality, and providing advanced analytical capabilities, Generative AI empowers businesses to make more informed decisions, improve operational efficiency, and gain deeper insights. As this technology continues to evolve, its impact on data management and analytics will undoubtedly grow, setting new standards for how businesses handle and leverage their data.
Footnotes:
Deloitte. (2024). Synthetic Data and Privacy Protection. Retrieved from Deloitte website.
Journal of Machine Learning Research. (2023). The Impact of Data Augmentation on Model Accuracy. Retrieved from JMLR website.
IBM. (2024). Enhancing Forecast Accuracy with AI-Driven Predictive Models. Retrieved from IBM website.
As the field of Generative AI continues to advance, its application to tabular data will become increasingly vital for businesses aiming to stay competitive and make data-driven decisions. Embracing this technology will enable organizations to unlock new opportunities and drive success in an increasingly data-centric world.
Comments