
Telecommunications companies face immense pressure to stay competitive in an evolving market. Predictive analytics offers powerful tools to anticipate customer needs, optimize operations, and enhance service delivery. Consulting with SynergyLabs provides the expertise necessary to implement these advanced analytical techniques effectively.
With the right predictive models, telecom providers can reduce churn, increase customer satisfaction, and enhance revenue streams. By leveraging data-driven insights, they can make informed decisions that lead to strategic improvements across various facets of their business. Engaging with SynergyLabs allows organizations to tap into tailored solutions that meet specific industry challenges.
The integration of predictive analytics into telecommunications can lead to substantial growth and efficiency. Industry leaders who prioritize these strategies are better equipped to navigate market complexities and ensure long-term success. Consulting experts like those at SynergyLabs can guide telecom companies in harnessing the full potential of their data analytics initiatives.
Fundamentals of Predictive Analytics in Telecommunications
Predictive analytics plays a crucial role in the telecommunications industry by enabling companies to analyze data trends and make informed decisions. This section explores the essence of predictive analytics, the significance of telecommunications data, and the associated benefits and challenges.
Understanding Predictive Analytics
Predictive analytics involves using historical data, statistical algorithms, and machine learning techniques to identify likely future outcomes. In telecommunications, it can forecast customer behavior, network performance, and market trends.
Telecom companies leverage predictive models to increase customer satisfaction and retention. By analyzing usage patterns, they anticipate when customers might churn and design targeted marketing strategies to retain them. The integration of predictive analytics into business processes allows for more proactive decision-making.
Role of Telecommunications Data
Data in telecommunications is vast and varied, including call records, customer demographics, billing information, and network performance metrics. Each type of data provides valuable insights that can enhance predictive models.
For instance, analyzing call detail records can reveal peak usage times, which helps in optimizing network resources. Subscriber demographic data contributes to targeted marketing efforts, while performance metrics ensure that service quality meets consumer expectations. This multi-faceted approach to data harnesses the power of analytics to drive operational improvements.
Benefits and Challenges
The advantages of implementing predictive analytics in telecommunications are significant. Companies can enhance operational efficiency, reduce churn rates, and optimize marketing campaigns.
However, challenges exist. Data privacy concerns must be addressed, especially with stringent regulations like GDPR. Additionally, the integration of various data sources can lead to compatibility issues, hindering the effectiveness of predictive tools. Companies need to invest in sophisticated analytics platforms and skilled personnel to maximize the potential of predictive analytics while navigating these complexities.
Key Applications and Use Cases
Predictive analytics plays a crucial role in telecommunications by enabling companies to make informed decisions. Its applications span various critical areas, leading to improved customer experiences and operational efficiencies.
Customer Churn Prediction
Customer churn prediction is essential for telecommunications providers aiming to retain subscribers. By analyzing customer data, including usage patterns and service interactions, predictive models identify individuals at risk of leaving. This allows companies to target at-risk customers with tailored retention strategies, such as special offers or loyalty programs.
Telecommunications firms can benefit from leveraging machine learning algorithms that incorporate demographic information and service usage metrics. By anticipating churn, they can reduce turnover and associated costs significantly.
Network Optimization
Network optimization focuses on enhancing the performance and efficiency of telecommunications networks. Predictive analytics evaluates historical and real-time data to identify potential congestion points and outages before they occur. By forecasting network demands, companies can allocate resources more effectively, ensuring high-quality service.
Additionally, predictive models help telecommunications providers deploy upgrades or infrastructure enhancements strategically. This proactive approach minimizes downtime and maximizes the user experience, leading to greater customer satisfaction.
Fraud Detection and Security
Fraud detection and security are paramount in the telecommunications industry due to potential financial losses and reputational damage. Predictive analytics identifies unusual patterns and anomalies in usage data that may signify fraudulent activities.
Through advanced algorithms, telecommunications companies can monitor accounts for red flags such as sudden spikes in usage or irregular billing patterns. By addressing fraud proactively, they can enhance their security measures and protect customer data and assets.
Revenue Assurance
Revenue assurance involves safeguarding a telecommunications company’s income by preventing revenue leakage. Predictive analytics helps identify discrepancies in billing, usage reporting, and customer transactions. Analyzing trends and patterns ensures that all services rendered are accurately billed, reducing financial losses.
This approach allows companies to investigate and rectify issues swiftly. Implementing predictive models enhances accuracy in revenue forecasting, contributing to healthier financial performance and better resource management.
Personalized Marketing and Customer Service
Personalized marketing and customer service harness customer insights to create tailored experiences. Predictive analytics evaluates customer preferences, behavior, and demographic information to develop targeted marketing campaigns. By understanding individual needs, telecommunications companies can boost engagement and conversion rates.
Furthermore, predictive models support proactive customer service by anticipating issues before they arise. By predicting service disruptions or customer inquiries, companies can address concerns promptly, leading to increased loyalty and satisfaction.
Data Management and Quality
Effective data management and maintaining high data quality are crucial for implementing predictive analytics in telecommunications. Proper handling of data ensures that insights generated are accurate and actionable, which directly impacts decision-making processes.
Data Collection
Data collection involves gathering information from various sources, including customer interactions, network performance metrics, and billing records. Telecommunications companies utilize both structured and unstructured data, which may include customer surveys, social media feedback, and device usage statistics.
Employing advanced tools like data scraping and API integration enhances data collection efficiency. Continuous monitoring of data sources ensures relevance and timeliness. Establishing robust collection protocols is essential to mitigate bias and ensure comprehensive coverage of relevant data points.
Data Storage and Processing
Data storage and processing methods are vital for supporting predictive analytics initiatives. Organizations typically rely on cloud storage solutions, which offer scalability and flexibility. Modern data warehouses can handle vast amounts of data, ensuring that information is accessible and secure.
Processing methods involve ETL (Extract, Transform, Load) procedures, where raw data is cleansed and structured for analysis. Implementing real-time data processing systems allows organizations to respond rapidly to trends or anomalies, thus enhancing operational efficiency and customer satisfaction.
Data Quality Issues
Data quality issues can significantly hinder predictive analytics efforts. Common challenges include missing data, duplicate entries, and inaccuracies that may skew results. Regular audits should be conducted to identify and rectify these issues promptly.
Employing data quality frameworks helps in assessing and maintaining consistency, completeness, and validity of data. Using automated tools for data validation aids in identifying errors quickly, ensuring that the data used for predictive analytics is reliable and trustworthy. Addressing data quality proactively enables better insights and strategic planning.
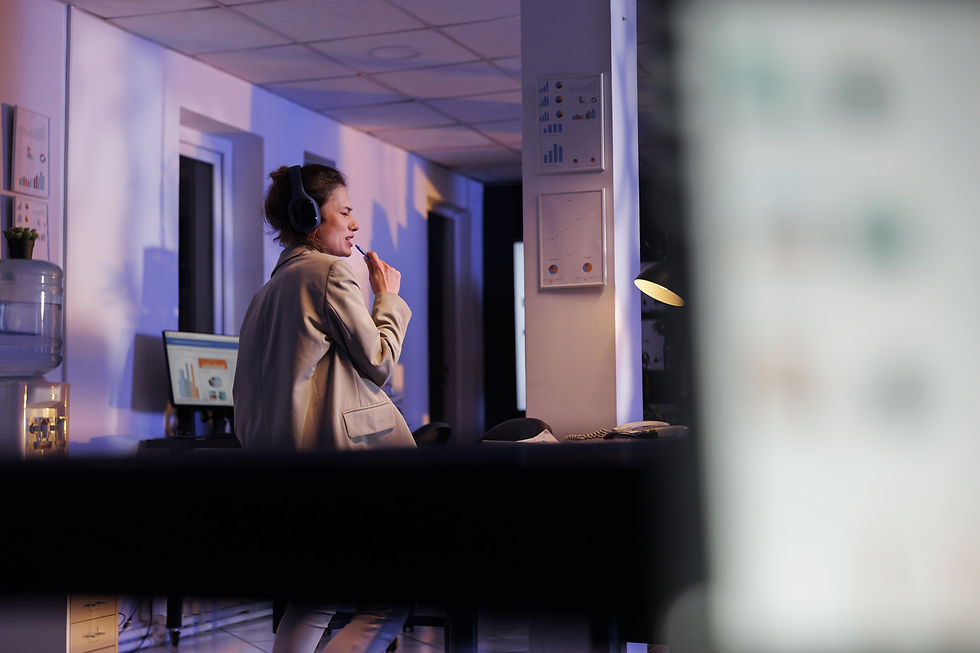
Predictive Modeling Techniques
Predictive modeling techniques play a crucial role in telecommunications, allowing companies to forecast customer behavior, optimize operations, and enhance service delivery. These techniques can be categorized into statistical models, machine learning algorithms, and ensemble approaches, each offering unique advantages.
Statistical Models
Statistical models are foundational in predictive analytics, relying on mathematical relationships to forecast outcomes. Common techniques include linear regression, logistic regression, and time series analysis.
Linear Regression: Used for predicting continuous outcomes, it establishes a relationship between variables. For example, it can predict churn rate based on customer usage metrics.
Logistic Regression: This is ideal for binary outcomes, such as customer retention or abandonment.
Time Series Analysis: Focuses on data points collected over time, crucial for identifying trends and seasonality in customer usage patterns.
These models are favorable for their interpretability and efficiency, often providing quick insights and reliable forecasts.
Machine Learning Algorithms
Machine learning algorithms offer advanced methods for predictive modeling, capable of capturing complex patterns in large datasets. Popular methods include decision trees, random forests, and support vector machines.
Decision Trees: These models split data into branches based on feature values, making them easily interpretable.
Random Forests: An ensemble of decision trees, they enhance accuracy and reduce overfitting by averaging multiple predictions.
Support Vector Machines (SVM): Effective for classification tasks, SVM identifies the optimal boundary between classes of data points.
These algorithms are particularly beneficial in telecommunications for segmenting customers and predicting behavior based on rich datasets.
Ensemble Approaches
Ensemble approaches combine multiple predictive models to improve accuracy and robustness. Techniques include bagging, boosting, and stacking.
Bagging: This method reduces variance by combining predictions from multiple models trained on random subsets of the data.
Boosting: Focuses on all models sequentially, where each new model pays attention to errors made by the previous one.
Stacking: Involves training a meta-model to combine the predictions of various base models.
Utilizing ensemble approaches can lead to significant performance improvements, making them invaluable for telecommunications companies aiming to leverage predictive analytics effectively.
Implementation Strategies
To successfully implement predictive analytics in telecommunications, organizations must focus on building internal capabilities, partnering with expert firms, and managing change effectively. These strategies ensure a well-rounded approach to integrating advanced analytics into business operations.
Building Internal Capabilities
Developing internal expertise is crucial for leveraging predictive analytics effectively. Organizations should invest in training programs to enhance employees' skills in data analysis, machine learning, and statistical methods. Workshops, online courses, and certification programs can ensure teams are equipped with the necessary knowledge.
Creating a dedicated analytics team can facilitate deeper insights and foster a culture of data-driven decision-making. This team should collaborate across departments to ensure that analytics initiatives align with organizational goals. Additionally, maintaining access to analytics tools and resources will support ongoing development and refinement of predictive models.
Partnering with Expert Firms
Speak to Synergy labs representatives to accelerate the implementation of predictive analytics. These firms bring extensive knowledge and experience, providing valuable insights into best practices and emerging trends. A strategic partnership enables organizations to leverage advanced technologies and methodologies without the steep learning curve.
Co-developed solutions address specific business challenges. This collaboration not only enhances capabilities but also streamlines processes and reduces time-to-market for analytics projects.
Managing Change in Organizations
Implementing predictive analytics often requires significant changes within an organization. To navigate this transition, effective change management strategies are essential. Leaders should communicate the benefits of analytics clearly to all stakeholders, emphasizing how it can improve decision-making and enhance customer satisfaction.
Fostering a culture that embraces data and analytics will facilitate smoother adoption. Engagement sessions, feedback loops, and pilot projects can help address resistance and demonstrate value. It is important to involve employees in the process, allowing them to contribute and adapt to new analytical tools.
Case Studies and Industry Insights
Telecommunications companies have increasingly adopted predictive analytics to enhance operations. Case studies reveal its effectiveness in various areas.
Example 1: A major telecom provider used predictive analytics to reduce churn rates. By analyzing customer behavior patterns, they identified at-risk customers and implemented targeted retention strategies. This led to a 15% reduction in churn.
Example 2: Another organization utilized predictive models to optimize network performance. By forecasting traffic demands, they improved resource allocation, resulting in a 20% decrease in network congestion.
Industry Insights
Market Growth: The predictive analytics market in telecommunications is expected to grow by 25% annually.
Customer Satisfaction: Companies implementing these technologies often report improved customer satisfaction scores, attributed to more personalized services.
Key Benefits:
Cost Reduction: Efficient resource use leads to significant cost savings.
Enhanced Decision-Making: Data-driven insights support better strategic planning.
Consulting firms like SynergyLabs provide expert guidance on implementing predictive analytics. They assist organizations in leveraging data effectively to achieve measurable results. This approach enables telecom companies to stay competitive and responsive in a dynamic market.
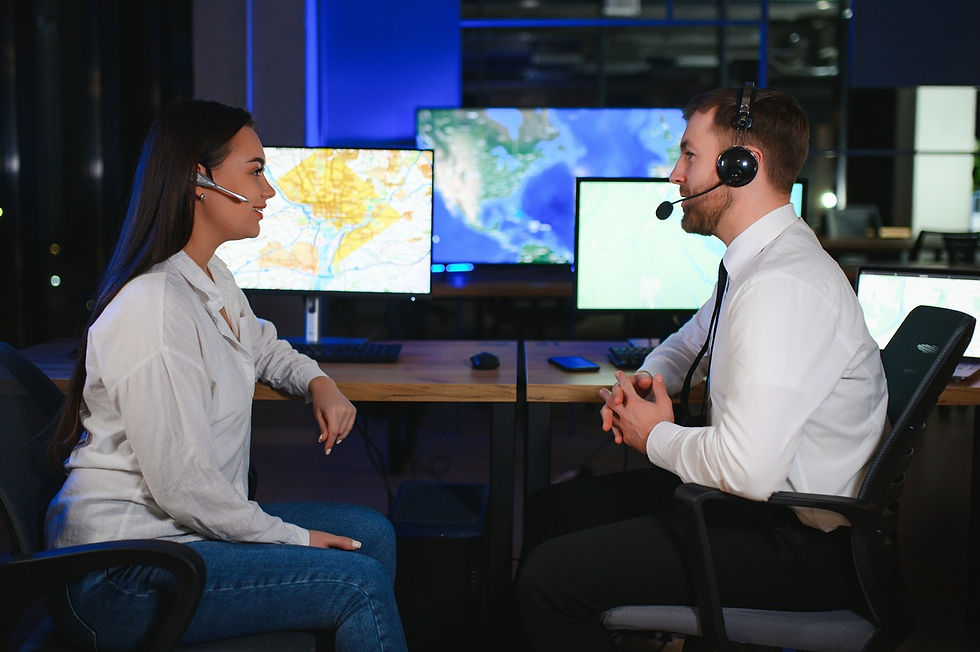
Future Trends and Innovations
As the telecommunications industry evolves, predictive analytics will play an increasingly pivotal role. Key innovations are expected to emerge in technology, regulatory frameworks, and network capabilities, reshaping how companies operate and deliver services.
Emerging Technologies in Predictive Analytics
The integration of machine learning and artificial intelligence into predictive analytics is revolutionizing telecommunications. These technologies enhance data processing capabilities, allowing service providers to analyze massive datasets in real time.
Natural language processing (NLP) tools are gaining traction for customer interaction analysis, predicting customer sentiments, and improving service quality. Additionally, advancements in cloud computing provide scalable resources that facilitate sophisticated analytics without infrastructure constraints.
Telecom companies can leverage these technologies for better demand forecasting, churn prediction, and enhanced customer experience, resulting in increased operational efficiency.
Impact of 5G and Beyond
The rollout of 5G networks significantly impacts predictive analytics in telecommunications. With greater bandwidth and lower latency, 5G enables real-time data collection from connected devices. This wealth of data can drive more accurate predictive models, resulting in better customer insights and personalized services.
Moreover, 5G’s capacity to handle billions of devices creates an opportunity for Internet of Things (IoT) analytics, enhancing operational efficiencies and enabling predictive maintenance. As organizations adapt to 6G and future network standards, they will harness more advanced analytics to optimize network performance.
Regulatory and Ethical Considerations
As predictive analytics becomes integral to telecommunications, regulatory and ethical factors must be addressed. The use of customer data, especially for predictive purposes, necessitates strict compliance with regulations such as the General Data Protection Regulation (GDPR).
Telecommunications providers must ensure transparency in data processing and implement robust cybersecurity measures. Ethical considerations include protecting customer privacy and preventing bias in predictive models.
Companies like SynergyLabs can provide guidance to navigate these complex regulatory landscapes and develop compliant analytics strategies.
Crafting a Telecommunications Analytics Roadmap
Creating a roadmap for telecommunications analytics is essential for guiding organizations through the complexities of predictive analytics. Three critical components include setting clear objectives, identifying resource and skill requirements, and operationalizing analytics insights.
Setting Clear Objectives
Establishing specific goals is fundamental in a telecommunications analytics roadmap. Objectives should align with both short-term and long-term business strategies. For instance, a company may aim to reduce churn rates or improve customer satisfaction scores.
Key performance indicators (KPIs) should be defined to measure success. These can include metrics such as Average Revenue Per User (ARPU) and Net Promoter Score (NPS). By outlining clear objectives, organizations can better align their analytics efforts with business outcomes.
Resource and Skill Requirements
Successful implementation of predictive analytics requires an assessment of available resources and needed skills. Organizations must evaluate existing technology infrastructures such as data management systems and software tools.
Additionally, the skill sets necessary for effective analytics should be identified. This may involve hiring data scientists, business analysts, and IT specialists. Training existing staff on new tools and methodologies is equally important to bridge any skills gaps.
Operationalizing Analytics Insights
To reap the benefits of predictive analytics, organizations must turn insights into actionable strategies. This involves integrating analytics into daily operations and decision-making processes.
They should develop a structured approach to incorporate findings into product development, marketing, and customer service. For instance, using predictive models to identify high-value customers allows for targeted marketing efforts. Regular updates and evaluations of the analytics process can ensure continuous improvement and adaptation to changing market demands.
Conclusion
Predictive analytics plays a crucial role in the telecommunications industry. Organizations can leverage data to enhance decision-making and operational efficiency.
By consulting SynergyLabs, businesses gain access to expert guidance in predictive analytics tailored for telecommunications. This collaboration enables companies to harness the full potential of their data.
Key benefits include:
Improved Customer Retention: Identifying at-risk customers and implementing targeted strategies.
Enhanced Service Quality: Predicting network issues before they affect users.
Cost Optimization: Making informed budget decisions based on predictive insights.
Investing in predictive analytics leads to informed strategies that drive growth. With expert support from SynergyLabs, telecommunications companies can stay competitive in a rapidly evolving market.
Comments