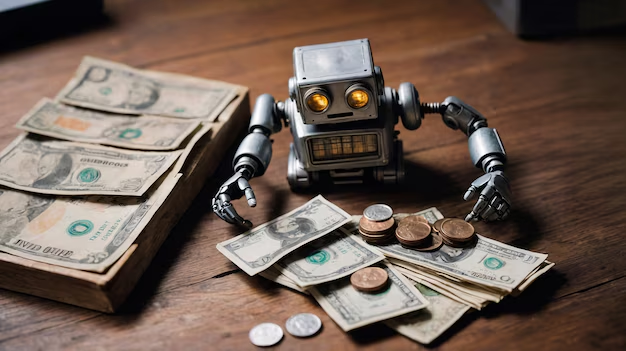
In recent years, generative AI has emerged as a powerful tool poised to reshape various industries, including investment banking. This advanced technology brings innovative solutions to the table, redefining how financial services operate. From automating routine tasks to enhancing decision-making processes, generative AI is changing the landscape of investment banking.
Understanding Generative AI in Finance

Defining Generative AI
Generative AI refers to a category of artificial intelligence that is capable of generating new data, content, or solutions based on the input data it receives. Unlike traditional AI, which focuses on pattern recognition and prediction, generative AI creates new possibilities, offering a fresh perspective in the financial sector.
Generative AI encompasses a range of technologies that include neural networks, deep learning, and reinforcement learning. These systems can autonomously create text, images, music, and even entire simulations. In finance, these capabilities translate into generating novel investment strategies, predictive models, and financial reports. By understanding the distinct functionalities of generative AI, financial professionals can leverage its full potential.
How Does Generative AI Work?
Generative AI uses algorithms and machine learning models to analyze vast amounts of data. By learning patterns and trends, it can generate new ideas, strategies, or predictions that add value to financial services. This process includes training models on historical data and fine-tuning them to adapt to current market conditions.
Algorithmic Foundations
At its core, generative AI relies on complex algorithms that enable it to process and interpret large datasets. These algorithms, such as Generative Adversarial Networks (GANs) and Variational Autoencoders (VAEs), allow AI to generate high-quality outputs by simulating human-like creativity. Understanding these foundational elements is crucial for deploying AI in finance effectively.
Data Processing and Analysis
The ability of generative AI to analyze massive datasets is pivotal in the financial industry. By sifting through historical and real-time data, AI systems can identify patterns and anomalies that might elude human analysts. This data-driven insight forms the basis for generating new financial models and strategies, enhancing the accuracy and relevance of predictions.
Model Training and Adaptation
Generative AI models require continuous training to remain effective. Financial markets are dynamic, with conditions that change rapidly. AI systems are therefore designed to adapt by learning from new data inputs, ensuring that their predictions and solutions remain current. This adaptability provides a significant advantage in the fast-paced world of finance.
The Role of Generative AI in Investment Banking
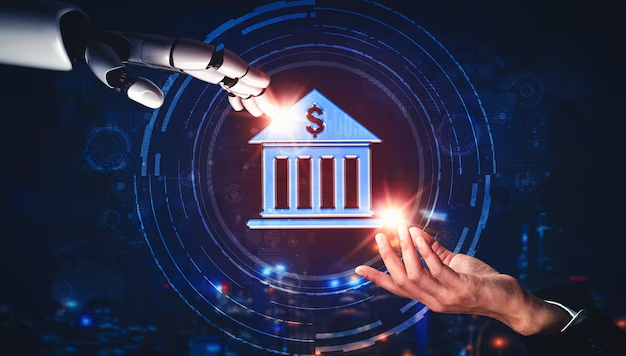
Investment banking involves complex activities such as mergers and acquisitions, risk management, and advisory services. Generative AI enhances these areas by providing more accurate analyses and forecasts, thus enabling bankers to make informed decisions quickly.
Mergers and Acquisitions
Generative AI assists in evaluating potential mergers and acquisitions by analyzing financial statements, market conditions, and strategic fit. By generating simulations and projections, AI offers bankers a clearer picture of potential outcomes, aiding in the negotiation and decision-making process.
Risk Management and Compliance
In the realm of risk management, generative AI offers advanced tools for assessing and mitigating risks. By generating risk models that account for various market scenarios, AI helps institutions prepare for volatility and regulatory changes. This capability is essential for maintaining compliance and safeguarding assets.
Strategic Advisory and Client Interaction
Generative AI enhances the advisory services offered by investment banks by generating detailed reports and strategic insights. These AI-driven analyses facilitate more informed client interactions, enabling bankers to provide customized advice and solutions that align with client objectives and market trends.
Key Benefits of Generative AI for Investment Banking
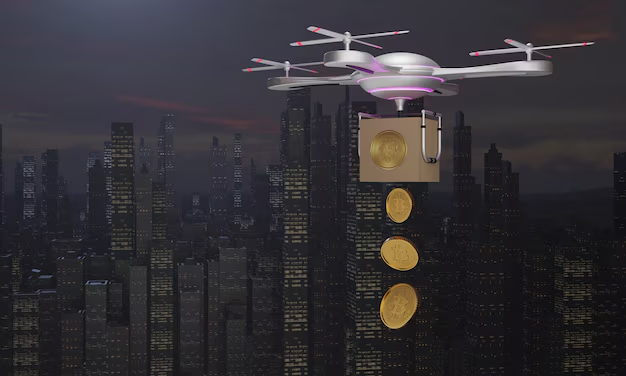
Enhanced Decision-Making
One of the most significant advantages of generative AI in investment banking is its ability to improve decision-making. By analyzing vast datasets, AI can identify patterns and predict market trends with greater accuracy than human analysts. This insight allows bankers to make strategic decisions based on data-driven evidence.
Data-Driven Insights
Generative AI excels in extracting actionable insights from complex datasets. By leveraging AI, investment banks can transform raw data into strategic intelligence, informing decisions on asset allocation, market entry, and capital management. This data-driven approach enhances the precision of financial decision-making.
Predictive Analytics
AI-driven predictive analytics allows banks to anticipate market movements and investor behavior. By generating forecasts based on historical data and current trends, generative AI supports proactive decision-making, enabling banks to seize opportunities and mitigate risks effectively.
Scenario Analysis and Simulation
Generative AI enables banks to conduct scenario analysis by simulating various market conditions and potential outcomes. This capability allows financial institutions to test strategies and assess the impact of different variables, leading to more robust and resilient decision-making processes.
Automation of Routine Tasks
Generative AI automates repetitive tasks that typically consume valuable time and resources. From data entry to transaction processing, AI can handle these tasks efficiently, freeing up human resources for more complex activities. This automation not only increases efficiency but also reduces the risk of errors.
Streamlining Operations
AI-driven automation streamlines operational workflows by eliminating manual processes. This efficiency reduces the time required for tasks such as document processing, compliance checks, and data validation, enabling banks to focus on strategic initiatives.
Error Reduction
By automating routine tasks, generative AI minimizes the risk of human error. This precision is particularly valuable in financial transactions, where mistakes can have significant consequences. AI ensures accuracy and consistency, enhancing the reliability of banking operations.
Resource Optimization
AI's automation capabilities allow banks to allocate human resources more effectively. By freeing employees from repetitive tasks, banks can redirect talent towards higher-value activities, such as client relationship management and strategic planning, maximizing productivity and impact.
Personalized Client Services
Generative AI offers the ability to personalize financial services for clients. By analyzing client data, AI can provide tailored investment advice, portfolio management, and risk assessment. This level of personalization enhances client satisfaction and strengthens relationships with financial institutions.
Tailored Investment Strategies
Generative AI analyzes individual client profiles to develop customized investment strategies. By considering factors such as risk tolerance, investment goals, and market conditions, AI delivers personalized recommendations that align with each client's unique financial objectives.
Enhanced Client Engagement
AI-driven personalization fosters deeper client engagement by delivering relevant insights and recommendations. This targeted approach enhances the client experience, building trust and loyalty while positioning banks as proactive partners in achieving financial success.
Dynamic Portfolio Management
Generative AI supports dynamic portfolio management by continuously monitoring market conditions and client preferences. AI systems can adapt investment strategies in real-time, ensuring that portfolios remain aligned with client goals and market dynamics.
Challenges in Implementing Generative AI

While the benefits of generative AI are clear, implementing this technology in investment banking presents certain challenges. Understanding these hurdles is crucial for a successful AI integration.
Data Privacy and Security
Financial institutions handle sensitive client data, making data privacy and security paramount. Ensuring that AI systems comply with regulations and protect client information is a major challenge that banks must address.
Regulatory Compliance
Investment banks must navigate complex regulatory landscapes to ensure that AI implementations comply with data protection laws. This includes adhering to regulations such as GDPR and ensuring that AI systems are transparent and accountable in their data handling practices.
Secure Data Management
AI systems require access to vast amounts of sensitive data, necessitating robust security measures. Banks must implement advanced encryption and authentication protocols to safeguard client information and prevent unauthorized access or data breaches.
Ethical Data Use
Ethical considerations in data use are critical for maintaining client trust. Banks must ensure that AI systems operate transparently and that data is used responsibly, avoiding biases and ensuring fairness in financial decision-making processes.
Integration with Existing Systems
Implementing generative AI requires integrating new technologies with existing systems. This process can be complex and may require significant investment in terms of time and resources. Ensuring seamless integration is essential to harness the full potential of AI.
Legacy System Compatibility
Banks often rely on legacy systems that may not be compatible with modern AI technologies. Overcoming this challenge requires careful planning and investment to ensure that AI systems can integrate smoothly with existing infrastructure, minimizing disruption.
Infrastructure Investment
Successful AI implementation may necessitate significant investment in technology infrastructure. This includes upgrading hardware, software, and network capabilities to support AI processes, ensuring that systems operate efficiently and effectively.
Change Management
Integrating AI into existing systems requires comprehensive change management strategies. Banks must engage stakeholders, provide training, and foster a culture of innovation to ensure that employees embrace AI technologies and understand their role in achieving business objectives.
Ethical Considerations
As AI takes on more decision-making roles, ethical considerations become important. Ensuring that AI systems operate transparently and fairly is crucial to maintaining trust with clients and stakeholders.
Bias and Fairness
Generative AI systems must be designed to avoid biases that could lead to unfair outcomes. This requires careful training and monitoring to ensure that AI models are objective and inclusive, supporting fair decision-making processes in financial services.
Transparency and Accountability
Transparency in AI operations is essential for building trust with clients and regulators. Banks must ensure that AI systems provide clear explanations for decisions and outcomes, enabling stakeholders to understand and assess the rationale behind AI-generated insights.
Human Oversight
Despite AI's capabilities, human oversight remains critical in financial decision-making. Banks must establish protocols for human intervention and review, ensuring that AI systems complement human expertise and uphold ethical standards in all interactions.
Real-World Applications of Generative AI in Investment Banking
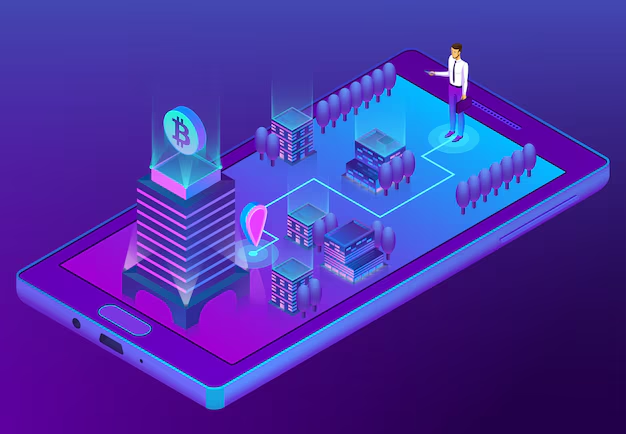
Risk Management
Generative AI enhances risk management by providing more accurate risk assessments. By analyzing historical and current data, AI can predict potential risks and suggest mitigation strategies, helping banks minimize financial losses.
Predictive Risk Modeling
AI systems generate predictive risk models that account for various market scenarios and uncertainties. These models enable banks to anticipate potential risks and develop proactive strategies to mitigate them, reducing the impact of adverse events.
Stress Testing and Scenario Analysis
Generative AI facilitates stress testing by simulating extreme market conditions and assessing their impact on financial portfolios. This analysis helps banks identify vulnerabilities and develop contingency plans to ensure resilience in volatile markets.
Real-Time Risk Monitoring
AI-driven risk management solutions offer real-time monitoring of financial markets and portfolios. By continuously analyzing data, AI systems can detect emerging risks and provide timely alerts, enabling banks to respond swiftly and effectively.
Fraud Detection
AI systems can detect fraudulent activities by identifying unusual patterns in transactions. This capability enables banks to respond quickly to potential threats, protecting both the institution and its clients.
Anomaly Detection
Generative AI excels in anomaly detection, identifying suspicious patterns and behaviors in financial transactions. By analyzing vast datasets, AI systems can flag potential fraud attempts, enabling banks to investigate and address threats promptly.
Real-Time Fraud Prevention
AI systems offer real-time fraud prevention by continuously monitoring transactions for irregularities. This proactive approach allows banks to intervene before fraudulent activities escalate, safeguarding client assets and institutional reputation.
Adaptive Security Measures
Generative AI supports adaptive security measures by learning from emerging fraud patterns. AI systems can adjust fraud detection algorithms based on new threats, ensuring that banks remain one step ahead of cybercriminals and evolving security challenges.
Portfolio Optimization
Generative AI assists in optimizing investment portfolios by analyzing market trends and predicting future performance. This insight helps bankers create balanced portfolios that align with their clients' financial goals.
Asset Allocation Strategies
AI-driven portfolio optimization involves generating asset allocation strategies that balance risk and return. By analyzing market data and client preferences, AI systems recommend allocations that optimize portfolio performance, enhancing investment outcomes.
Market Trend Analysis
Generative AI provides in-depth market trend analysis, offering insights into emerging opportunities and risks. This analysis helps bankers adjust portfolio strategies to capitalize on market movements and achieve client objectives.
Performance Forecasting
AI systems generate performance forecasts that predict future portfolio returns based on historical data and market conditions. These forecasts enable bankers to set realistic expectations and make informed decisions about portfolio adjustments and rebalancing.
The Future of Generative AI in Investment Banking
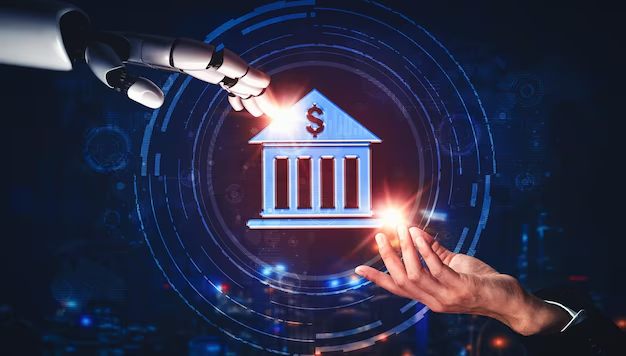
The future of investment banking is intertwined with the advancements in generative AI. As AI technology continues to evolve, its role in financial services will expand, offering even more innovative solutions and opportunities for growth.
Continuous Learning and Adaptation
Generative AI systems are designed to learn continuously, adapting to new data and changing market conditions. This adaptability ensures that AI remains relevant and effective, providing banks with a competitive edge in a rapidly changing environment.
Dynamic Learning Algorithms
AI systems employ dynamic learning algorithms that enable continuous improvement and adaptation. By learning from new data inputs and market developments, AI systems refine their models and predictions, ensuring sustained accuracy and relevance.
Market Responsiveness
Generative AI's ability to adapt to market changes enhances its responsiveness to emerging trends and opportunities. This agility allows banks to remain competitive by quickly adjusting strategies and offerings to align with evolving client needs and market conditions.
Innovation and Growth
The continuous learning capabilities of AI systems foster innovation and growth within financial institutions. By leveraging AI to explore new business models and services, banks can unlock new revenue streams and expand their market presence.
Collaboration Between Humans and AI
The future of AI in investment banking lies in the collaboration between human expertise and AI capabilities. By combining the analytical power of AI with human intuition and experience, banks can offer unparalleled services and achieve better outcomes for their clients.
Complementary Skill Sets
AI systems complement human skill sets by handling complex data analysis and pattern recognition tasks. This collaboration allows human professionals to focus on strategic thinking, creative problem-solving, and relationship-building, enhancing overall service delivery.
Enhanced Decision-Making
The synergy between humans and AI enhances decision-making processes by integrating data-driven insights with human judgment and intuition. This collaboration ensures that financial decisions are informed, balanced, and aligned with client objectives and values.
Building Client Trust
By leveraging AI to enhance service quality and decision-making, banks can build trust with clients by demonstrating their commitment to innovation and excellence. This trust is vital for establishing long-term relationships and driving client satisfaction and loyalty.
Conclusion
Generative AI is transforming investment banking by enhancing decision-making, automating routine tasks, and personalizing client services. While challenges exist, the benefits of AI integration are undeniable. As financial institutions embrace this technology, they position themselves at the forefront of innovation, ready to navigate the complexities of the modern financial landscape. The journey towards AI-driven banking is just beginning, and the possibilities are limitless.
Generative AI's transformative impact on investment banking is poised to redefine the industry, offering opportunities for enhanced efficiency, innovation, and client engagement. As banks continue to explore and integrate AI technologies, they will unlock new pathways to growth and success in an increasingly competitive and dynamic market.
Comments